Synthetic Data / Digital Twin
By generating synthetic data, Ceyeborg can simulate your detection problem thousands of times, incorporating diverse variations and scenarios, before training a neural network specifically tailored for your facility. This extensive replication ensures comprehensive coverage of potential edge cases and expected defects, providing a robust training foundation. Additionally, this approach mitigates privacy concerns associated with using extensive real data for training, as synthetic data can be employed to protect sensitive information.
Going beyond synthesizing data, digital twins can be utilized to virtually plan and integrate our custom in-line quality assurance hardware stations into your production line. This method allows for precise hardware adjustments and optimization without the need for costly prototyping and redesign iterations.
Dive deep into developer mode >
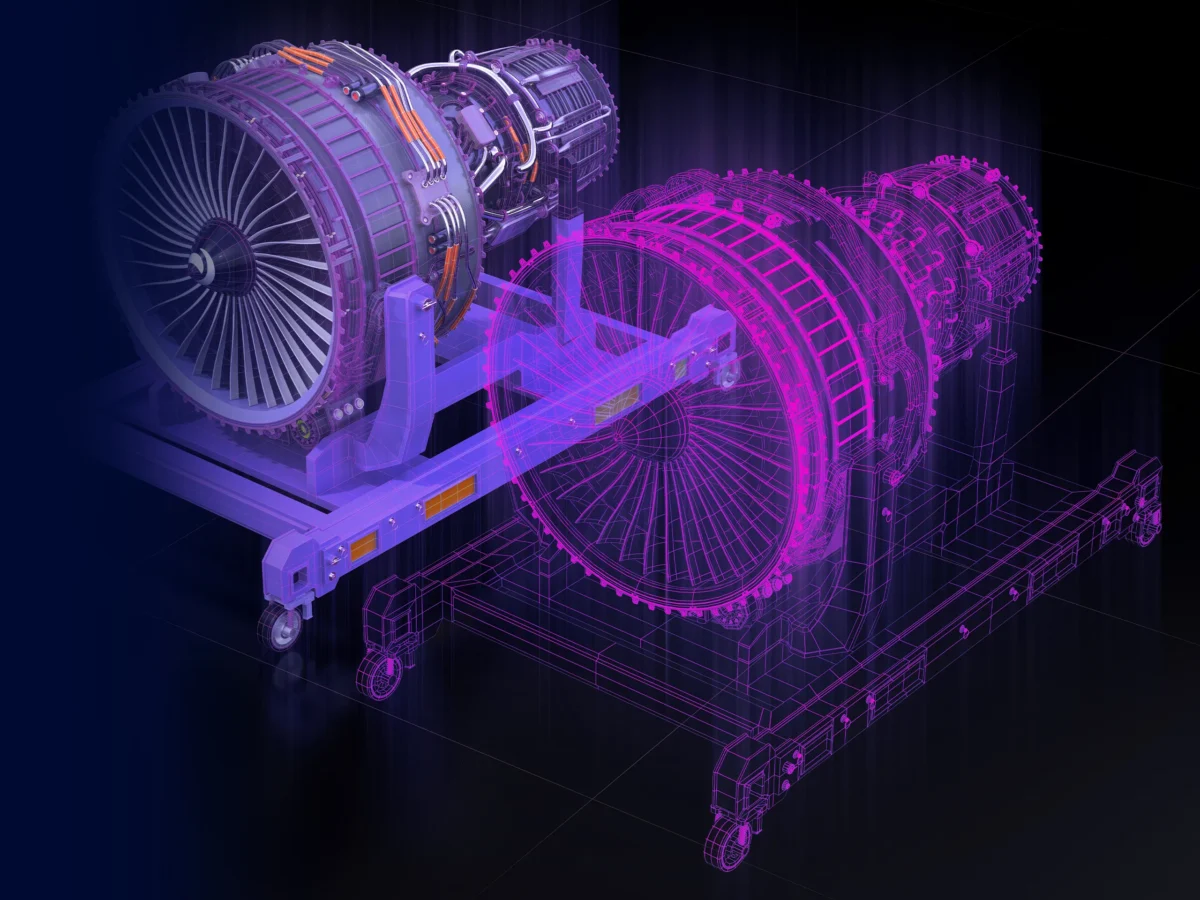
Synthetic Data / Digital Twin
Ceyeborg leverages advanced techniques in generative adversarial networks (GANs) and style transfer to produce high-fidelity synthetic data. For instance, style transfer can be used to generate diverse training images by transferring the style of one set of images onto the content of another. An example of synthetic data generation using style transfer is the creation of anomaly detection datasets. Suppose the real-world occurrence of defects or anomalies is infrequent. In this scenario, Ceyeborg’s tools can generate synthetic images of anomalies by blending the characteristics of known defects with normal operation images. This helps in training the AI models to accurately detect and classify such anomalies even when real-world examples are scarce.
Beyond generating synthetic data, the use of digital twins enables precise planning and modeling of quality inspection stations, guaranteeing that they are correctly configured to house sensor setups, custom PCBs, and AI inference stations. By simulating various scenarios and stress-testing the system, we can identify and mitigate potential issues, optimize system performance, and ensure seamless integration with existing workflows. This proactive approach not only reduces the need for costly adjustments and iterations during deployment but also enhances the reliability and efficiency of the inspection stations from the outset.
< BACK TO MANAGEMENT MODE
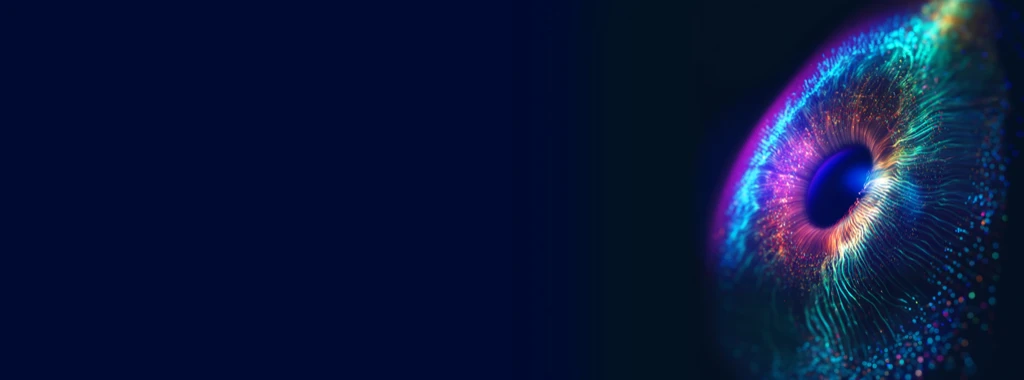
Dive deep into
developer mode
Back to
management mode